By Motivation When designing algorithms we want them to be as flexible as possible such that they can solve as many problems as possible. To solve a specific family of problems well, finding well-performing hyperparameter configurations requires us to either use extensive domain knowledge or resources. The second point is especially true if we […]
Our seven 2019 papers on neural architecture search (NAS)
By Neural Architecture Search (NAS) is a very hot topic in AutoML these days, and our group is very actively publishing in this area. We have seven NAS papers in 2019, which may make us one of the world’s most active groups in NAS (only closely surpassed by a small company called Google ;-). Here […]
RobustDARTS
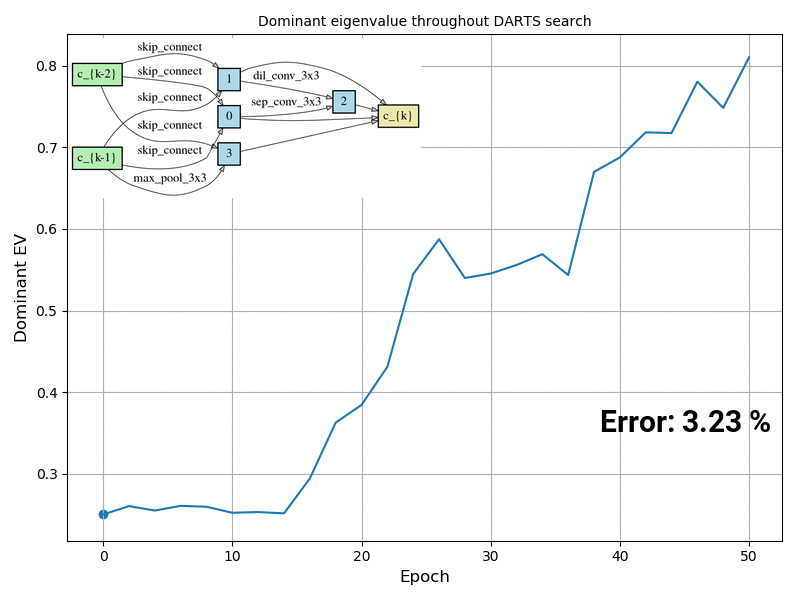
By Understanding and Robustifying Differentiable Architecture Search Optimizing in the search of neural network architectures was initially defined as a discrete problem which intrinsically required to train and evaluate thousands of networks. This of course required huge amount of computational power, which was only possible for few institutions. One-shot neural architecture search (NAS) democratized this […]
Best Practices for Scientific Research on Neural Architecture Search
By Neural architecture search (NAS) is currently one of the hottest topics in automated machine learning (see AutoML book), with a seemingly exponential increase in the number of papers written on the subject, see the figure above. While many NAS methods are fascinating (please see our survey article for an overview of the main trends […]
AutoDispNet: Improving Disparity Estimation with AutoML
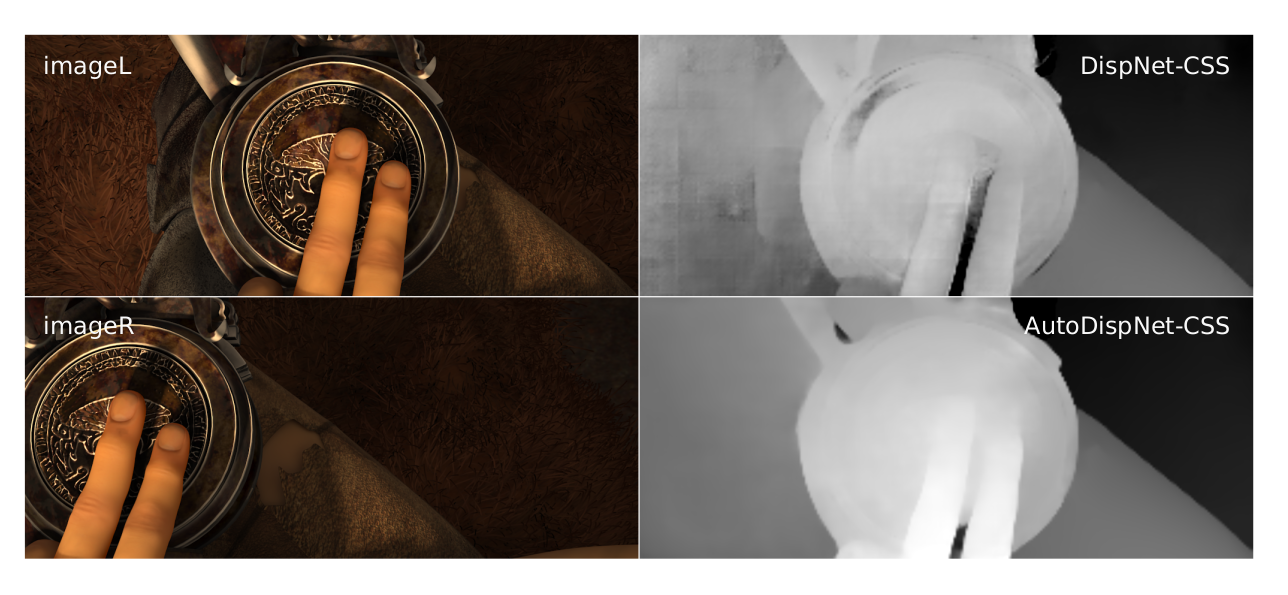
By Compared to the state of computer vision 20 years ago, deep learning has enabled more generic methodologies that can be applied to various tasks by automatically extracting meaningful features from the data. However, in practice those methodologies are not as generic as it looks at first glance. While standard neural networks may lead to […]
LEMONADE: Efficient Multi-objective Neural Architecture Search with Network Morphisms
By Most work on neural architecture search (NAS, see our recent survey) solely optimizes for one criterion: high performance (measured in terms of accuracy). This often results in large and complex network architectures that cannot be used in real-world applications with several other important criteria including memory requirement, energy consumption and latency. The other problem […]
NAS-Bench-101: Towards Reproducible Neural Architecture Search
By Much work in neural architecture search (NAS) is extremely compute hungry — so compute hungry that it hurts progress and scientific rigor in the community. When individual experiments require 800 GPUs for weeks nobody in academia can meaningfully join the community, and even in companies with huge compute resources nobody thinks of repeating their […]
Automatic Reinforcement Learning for Molecular Design
By In reinforcement learning (RL), one of the major machine learning (ML) paradigms, an agent interacts with an environment. How well an RL agent can solve a problem, can be sensitive to choices such as the policy network architecture, the training hyperparameters, or the specific dynamics of the environment. A common strategy to deal with […]
BOHB: Robust and Efficient Hyperparameter Optimization at Scale
By Machine learning has achieved many successes in a wide range of application areas, but more often than not, these strongly rely on choosing the correct values for many hyperparameters (see e.g. Snoek et al., 2012). For example, we all know of the awesome results deep learning can achieve, but when we set its learning […]
We did it again: world champions in AutoML
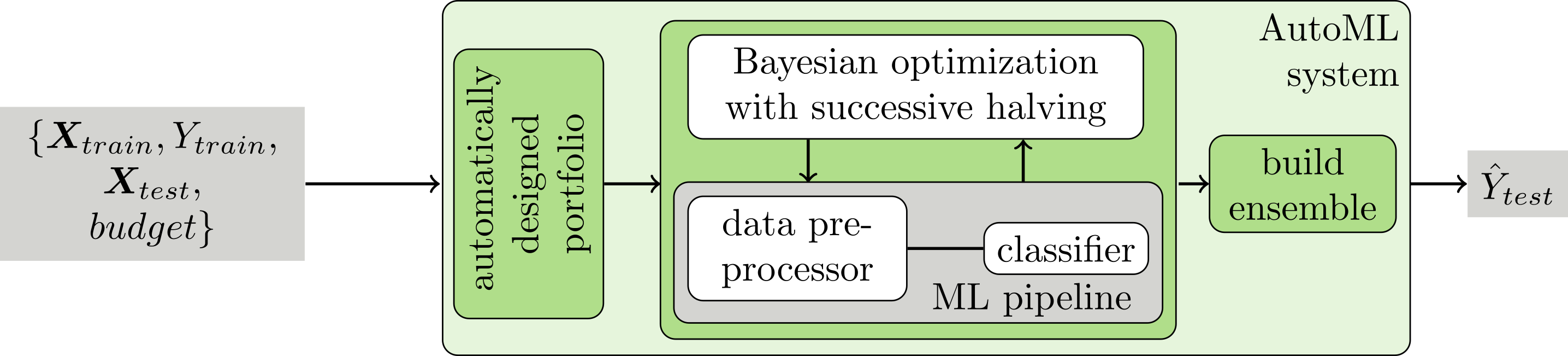
By Our ML Freiburg lab is the world champion in automatic machine learning (AutoML) again! After winning the first international AutoML challenge (2015-2016), we also just won the second international AutoML challenge (2017-2018). Our system PoSH-Auto-sklearn outperformed all other 41 participating AutoML systems.